D5.3: Toolkit for Data Sharing
Based on the review of literature on, and real-life challenges to data sharing (the latter informed by a series of conversations with outstanding researchers in CLS), this Wiki provides readily comprehensible and easily implementable recommendations and templates organized along the lines of Research Data Life Cycle. It covers research good practices in the context of CLS corpora and data with regard to:
- planning and designing data
- creating and collecting data
- preparing and enriching data
- preserving and publishing data
- reusing data
The wiki, distributed under Licence CC 0 4.0, addresses complex interactions between CLS researchers and other actors involved in data stewardship (data officers, data providers, technical officers, etc.). In line with the general character of the CLS INFRA project, whose participants attend to the needs of CLS researchers, we adopt the perspective of a scholar in their negotiations with institutions rather than representing an institutional point of view.
The wiki will be updated according to the evolution of Open Science policies and culture, in particular data sharing, while remaining a part of an infrastructural European framework for CLS.
Ch 1: Challenges to Data Sharing
This chapter highlights the reasons data sharing is increasingly recognised as a necessary condition for scientific innovation, and identifies challenges to sharing data.
Ch 2: Researchers' Voices
Five in-depth interviews with researchers informed this wiki. Click their names to read the full text of each interview.
Ch 3: Good Practices along the Research Data Life Cycle
Key practice: FAIR Data and Research Data Lifecycle
Author: Carolin Odebrecht
The FAIR Guiding Principles (Wilkinson et al. 2016) state that (meta)data should be Findable, Accessible, Interoperable, and Reusable. FAIR represents the interface between the high-level goals of research integrity and openness on the one hand and the process model research data lifecycle, on the other, developed within the context of research data management. This wiki section will explain the connections between the values and the practices as well as provide examples from the CLS domain of addressing both kinds of issues.
- 1st: Planning and Designing Data
- 2nd: Creating and Collecting Data
- 3rd: Preparing and Enriching Data
Author: Carolin Odebrecht
Firstly, CLS data typically require a careful corpus design reflecting for example the preservation conditions of sources and their genre see CLS INFRA’s Survey of Methods (Schöch, Dudar, and Fileva 2023) and register (Biber and Conrad 2019) identification, authorship attribution (Schöch, Dudar, and Fileva 2023), and the evaluation or classification of textual material with regard to, e.g., cultural, social, and literary contexts, traditions, and canonicity in specific CLS domains. In this section, we present three types of corpus design: Designed Corpus, Opportunistic/Growing/Dynamic Corpus and Edition.
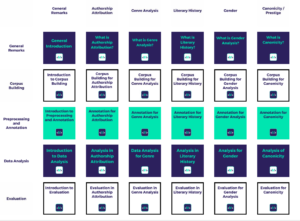
Author: Michał Mrugalski
Nothing else has the same impact on research findings in CLS as collecting / creating data according to a corpus design. At this stage, researchers can control a wide range of variables as their manipulation of these variables ultimately result in varied outcomes.
Conducive to the openness and the reproducibility of research, it is recommend that CLS researchers from the outset regard the process of collecting and creating data (corpora) as producing an artifact that can be reused by other researchers (Harrower 2020, 9).
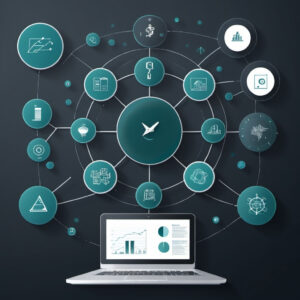
Author: Michał Mrugalski
Data preparation and enrichment cannot be easily separated from the following step in the life cycle of data, data analysis (discussed in Exploring and Analysing Data). Since data processing is typically the first step of data analysis, the way in which we approach a corpus with a research question and hypothesis will determine how the data is prepared.
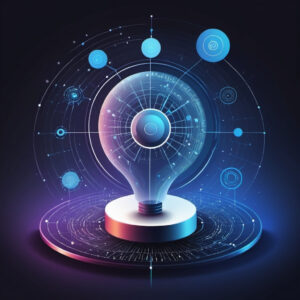
- 4th: Exploring and Analysing Data
- 5th: Preserving and publishing data
- 6th: Reusing Data
Author: Michał Mrugalski
The act of analyzing or evaluating data can be characterized as validating hypotheses on corpora in relation to population or, in more exploratory approaches, testing preliminary insights into data patterns, e.g. observing how corpus elements cluster with regard to some predetermined features. Analysis is done with an eye toward a result. It aims at extracting relevant information from data. Data should therefore become as a result of the analysis interpretable information in a human-readable form, such as a report, table, visualization, etc. Or, to put it differently, computing turns data into information interesting and intelligible for humans.
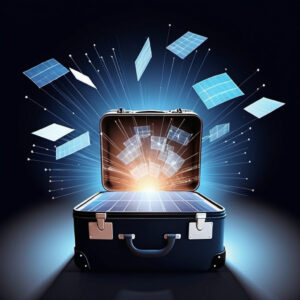
Author: Carolin Odebrecht
The preservation and publication of CLS data represents the crucial step in the research data lifecycle for data users because it makes the results of the previous steps available for re-use. Publication enables data creators to publish data sets (corpora, collections, editions) in order to follow good research practices that require validation, reproducibility, and citability of research data (cf. FAIR Data and Research Data Lifecycle). Data preservation is typically done by publication in a trustworthy repository.
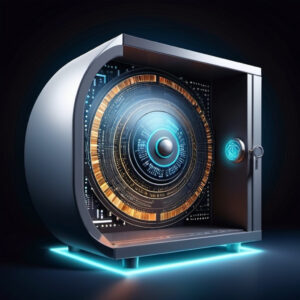
Author: Michał Mrugalski
The reuse of data lies at the core of the FAIR-ness and Open Science. Reusing data goes both ways. While reusing somebody else’s data, researchers are encouraged to design their own data reuseable.
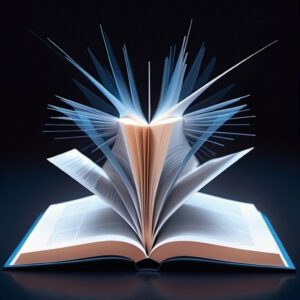